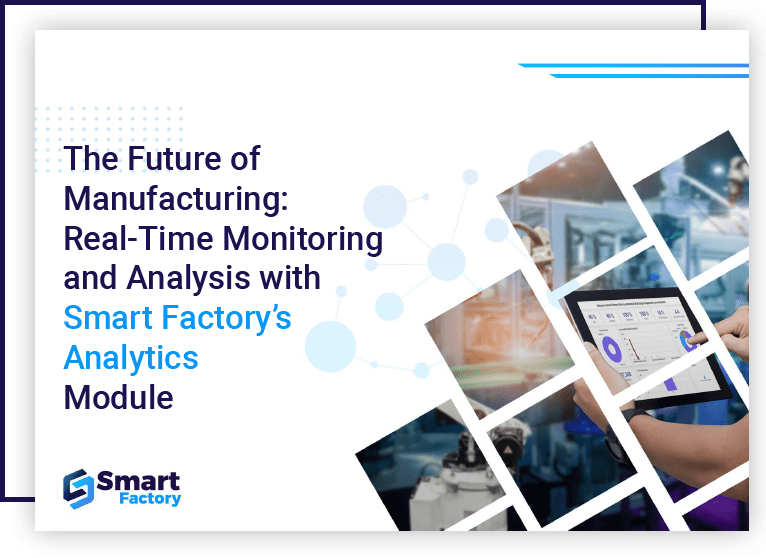
Power Up Your Production Processes With Smart Factory's Dynamic Analytics Module
Utilizing Predictive Analytics In The Manufacturing Sector
Primary Benefits of Real-Time Monitoring
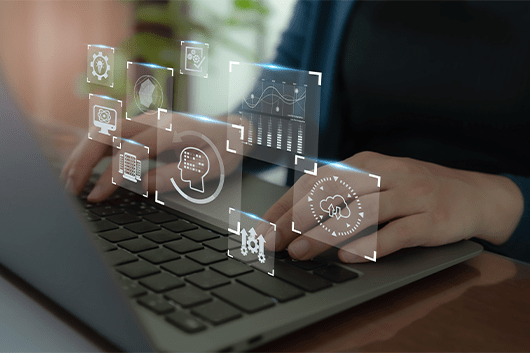
Optimal Decision-Making
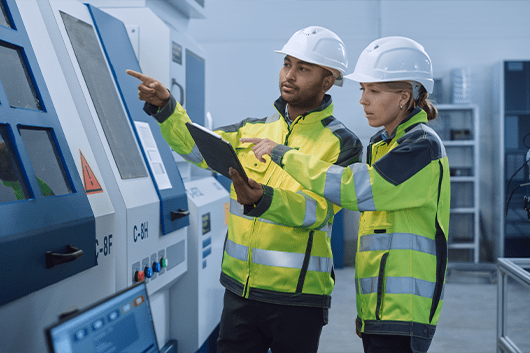
Increased Efficiency in Operations
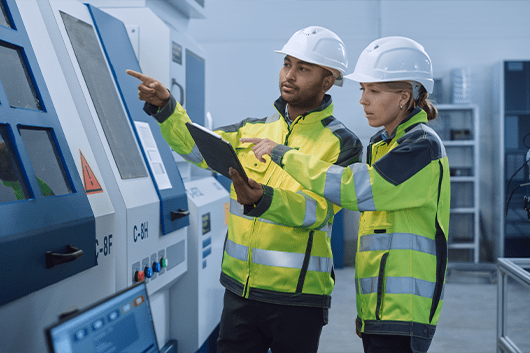
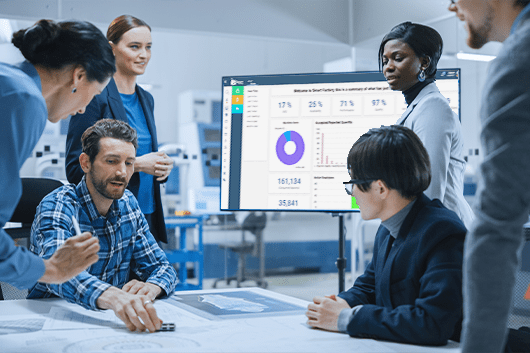
Preventive Identification and Handling of Problems
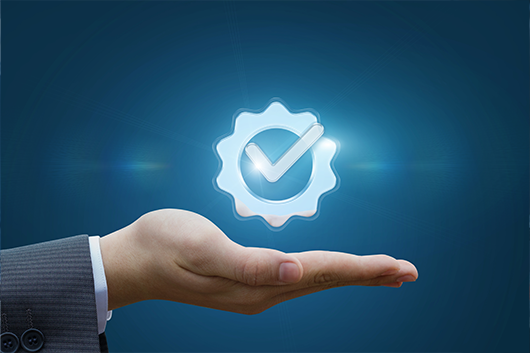
A Higher Level of Customer Satisfaction
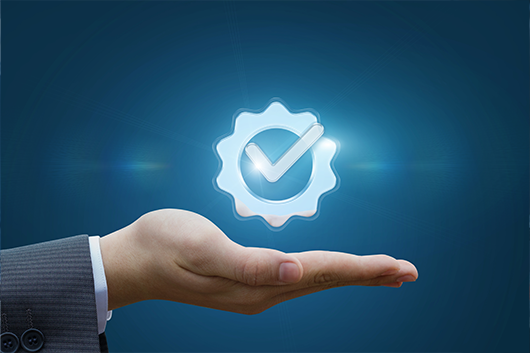
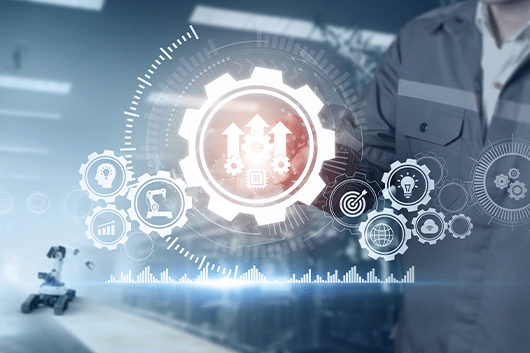
The Advantage Over Competitors
Essential Elements of Predictive Analytics

Gathering Data
Predictive analytics begins with the collection of high-quality data, a task made easy in the industrial sector. The Internet of Things (IoT) sensors play a significant role, linking manufacturing systems to the Internet and enabling the collection of data from actual events. This data serves as the foundation for using machine learning models to make predictions.

Preprocessing Data
Manufacturers have made significant investments in data gathering. However, to fully utilize this data, the raw data must undergo preparation so that machine learning algorithms can effectively use it. Correct organization of the data is necessary, and issues such as inconsistent formatting, missing numbers, outliers, and noise must be addressed for machine learning readiness. This crucial procedure, known as "data preprocessing," is essential for producing accurate and performative predictions.

Model Building
Following the gathering and preparation of data, the next stage involves developing the model. Machine learning algorithms are trained to generate predictions on test data that have not been seen before. In this process, algorithms identify patterns and correlations between variables. The selection of the most suitable model depends on the type of problem being addressed and the available data.

Model Assessment
Analyzing the model's performance in relation to the test data allows for a quantitative determination of its effectiveness. This stage also provides a benchmark for comparison to ascertain which trained model method is best suited for the situation. Metrics such as precision, recall, and F1 score for each model algorithm are used to evaluate its accuracy and dependability. These measures can be derived using the k-cross validation approach or the holdout method.
Manufacturing Applications for Predictive Analytics
Predictive Maintenance
Optimization of Facilities
Forecasting Demand
Predictive Analytics' Advantages in Manufacturing
Diminished Costs
Making Decisions Based on Data
A Higher Caliber of Products
Predictive Analytics Implementation Challenges
Gathering of Data
Data Retention
Insufficient Knowledge of Machine Learning
Predictive Analytics's Place in the Future of Manufacturing
Predictive Analytics Available for Self-Usage
Digital Twins
Greater Focus on Sustainability
What Makes Us the Best?
Smart Factory MOM Solution: A Cut Above the Rest

Real-Time Monitoring Excellence
Our Smart Factory MOM solution offers a state-of-the-art real-time monitoring system that allows businesses to keep a pulse on their operations round the clock. With a user-friendly interface and robust functionality, our platform ensures that crucial data is at your fingertips when you need it the most.

Predictive Analytics Mastery
Our solution goes beyond hindsight to provide foresight. By utilizing machine learning models trained on vast datasets, businesses can anticipate trends, identify potential issues, and make data-driven decisions that set them apart from the competition.

Operational Optimization
We understand the challenges faced by manufacturing businesses in optimizing their operations. Our Smart Factory MOM solution addresses these challenges head-on, offering tools and features that enable facilities to run more efficiently, reduce downtime, and enhance overall productivity.

Sustainability Focus
In alignment with the growing global emphasis on sustainability, our solution incorporates features that promote resource efficiency and environmental responsibility. By adopting our Smart Factory MOM solution, businesses can contribute to a greener and more sustainable future.